One of the most accessible and practical AI trends for businesses is the rise of AI agents.
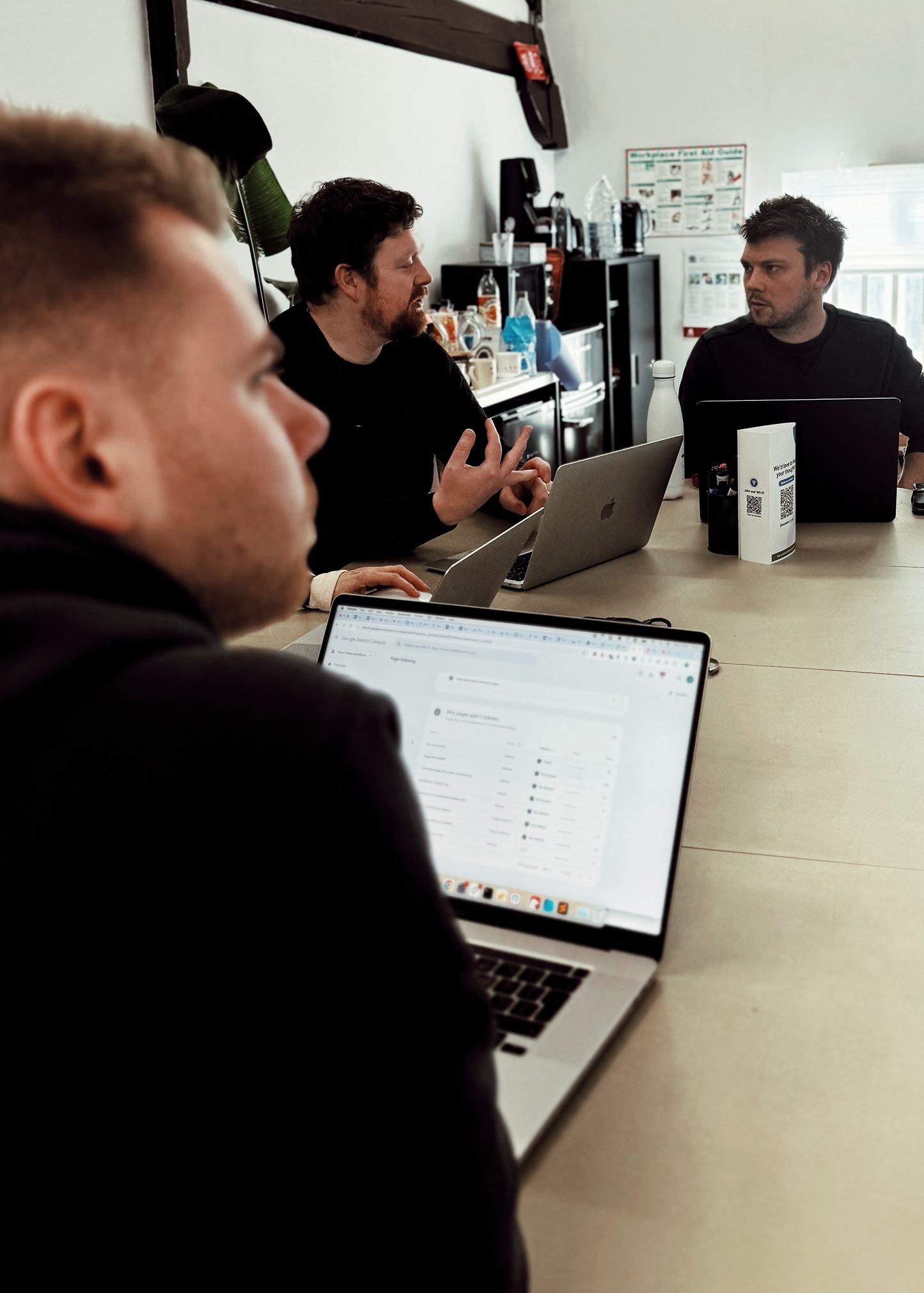
If you’ve spent more than 15 minutes on LinkedIn in the past week, you’ve probably seen a post about AI. It’s one of the most talked-about topics right now, and for good reason. One of the most accessible and practical AI trends for businesses is the rise of AI agents.
Unlike some of the more abstract or high-barrier applications of AI, AI agents are relatively easy to adopt. They don’t require huge investment or deep AI expertise. That’s why they’re a great way for businesses to start using AI in a meaningful, results-driven way.
What is an AI agent?
An AI agent is a system that can complete tasks autonomously based on goals you give it. Think of it as an assistant that doesn’t just follow instructions step-by-step but can understand context, decide how to act, and adapt as needed.
Unlike traditional automation (like if-this-then-that rules), AI agents can:
- React to new data or changes in real time
- Plan a series of actions to achieve an outcome
- Collaborate with APIs, apps, or other agents
- Learn and improve from outcomes
Some agents are based on large language models like GPT, while others are built on more specific frameworks. But they share a common aim: to act as autonomous problem-solvers.
Why are AI agents useful?
AI agents can be transformative for teams who are stretched, handling repetitive tasks, or trying to scale without adding more headcount. Here’s why they matter:
Automate repetitive, time-consuming tasks: agents can take over manual processes that slow your team down, such as checking inboxes, pulling reports, or creating proposals.
Reduce human error: by following a consistent set of logic, agents can avoid common mistakes and ensure the same level of output every time.
Free up your team for deeper work: instead of spending hours on low-impact (and frankly boring) admin, your team can focus on strategy, creativity, or client relationships.
Handle complex workflows: AI agents can chain multiple steps together, handle conditional logic, and even switch strategies mid-task if something changes.
Work 24/7: they don’t take breaks. They don’t get tired. They can run checks, monitor data, and respond to issues outside of working hours.
What’s the best way to start using an AI agent?
You don’t need to dive into a full AI transformation to get started. In fact, we’d recommend the opposite: start small, with a clear goal.
Here’s a simple framework:
Identify a repetitive task
Think about tasks your team does weekly or daily. Do any of them follow a clear process? Would it help if they happened faster or automatically?
Check for available tools or integrations
You don’t always need to build from scratch. Many no-code and low-code platforms (like Zapier, Make, or AI-specific platforms like AutoGPT, CrewAI, and LangChain) now support agent-based workflows.
Start with a test environment
Let the agent run in parallel with your current process. Monitor its decisions. Refine the logic or prompts if needed.
Measure the results
Time saved, tasks completed, error reduction, client response time – track the difference it makes so you can learn from what you have created so far, improve it or make further investment into the agent or create new ones.
Scale or iterate
Once you have one successful agent, think about where else it could help. Or combine agents into more complex flows.
How we’ve used AI agents
We’ve tested and implemented a number of agents internally, especially where we wanted to reduce manual overhead or improve response times. Here are some examples that other businesses could easily replicate:
Support inbox monitor: we have an agent that checks a shared inbox, drafts replies based on the message context, and flags urgent items to the right team member. It doesn’t replace our team, it accelerates their response.
Website performance monitoring: another agent runs checks on page speed and uptime, flags anomalies, and compiles a simple weekly report with a clear summary. No more waiting to log in to multiple dashboards.
Ticket triage and prioritisation: for our internal task management system, we have an agent that reviews ticket content and urgency, tags tasks accordingly, and nudges team members about time-sensitive issues.
Combined, these agents save us hours every week and have improved how quickly we spot and respond to client needs. They’ve also created a buffer, giving us breathing room during busier weeks.
Common challenges and how to overcome them
AI agents aren’t magic. Like any tool, they need the right setup. Here are some things to watch out for:
Over-engineering – don’t start with a complex process. Begin with a well-defined task and iterate from there.
Poor data inputs – if your agent is pulling from inconsistent, messy, or unclear data, its output will be just as poor. Clean data is essential.
Lack of monitoring – agents should be reviewed, eespecially early on. You need to trust but verify.
Unclear goals – know exactly what success looks like. Is it saving time? Reducing error? Improving output?
Summary
AI agents are not a fad, they’re a practical tool with real-world value. For most businesses, they offer the easiest and most immediate way to benefit from AI without deep technical investment.
Whether it’s speeding up internal tasks, improving accuracy, or helping your team respond faster, agents can deliver measurable gains.
Start with one problem. Solve it well. Then build from there.
It’s not about replacing people. It’s about giving your team better tools and more time to do what they do best.